ByteDance OmniHuman-1: A powerful framework for realistic human video generation
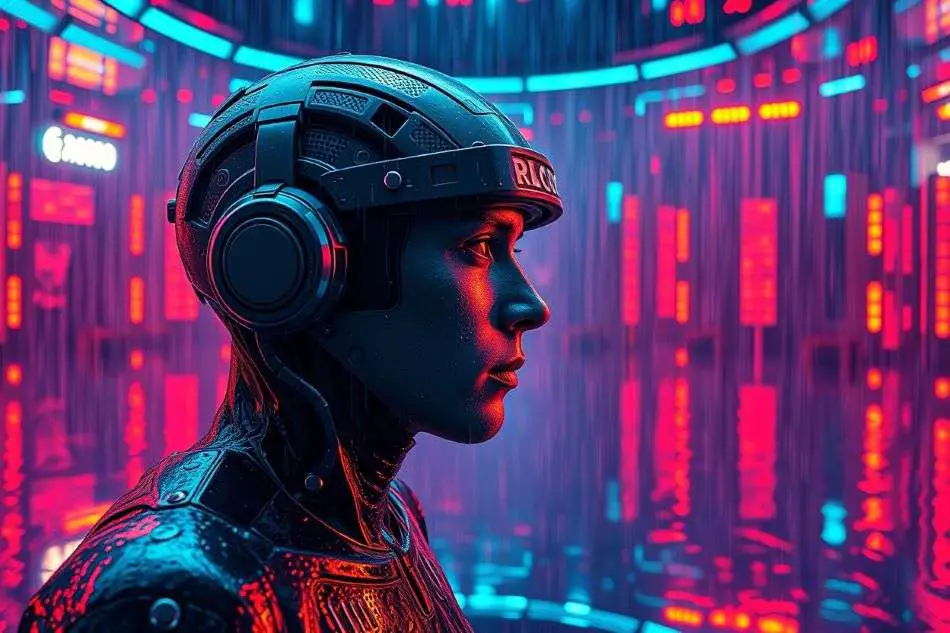
ByteDance has introduced OmniHuman-1, an AI model that generates realistic human videos using a single image and motion signals. OmniHuman-1 uses a Diffusion Transformer (DiT) architecture and an omni-conditions training strategy to fuse multiple input types—audio, video, and pose—to create full-body human animations. This breakthrough addresses longstanding challenges in AI-driven human animation and offers new practical applications for digital media and content creation.
In this article, we explore the technical details, performance benchmarks, and real-world use cases of this mind blowing breakthrough.
Key Takeaways
- Multimodal Input: OmniHuman-1 uses audio, video, and text to drive realistic full-body animations from a single image.
- Diffusion Transformer Architecture: The model leverages a DiT-based framework to integrate diverse motion signals and generate natural gestures and head movements.
- Omni-Conditions Training: It combines strong and weak motion conditions to enhance data diversity and improve motion realism.
- Competitive Benchmarks: The system demonstrates strong performance in lip-sync accuracy, gesture expressiveness, and hand keypoint confidence compared to leading models.
- Wide-Ranging Applications: The model benefits virtual avatars, digital storytelling, game development, and AI-assisted filmmaking through its flexible and scalable design.
OmniHuman-1: a new approach to human animation
In early 2025, ByteDance proposed OmniHuman-1 as an end-to-end multimodality framework. Unlike previous methods that primarily focused on facial or static body animations, this one generates dynamic, full-body human videos using a single reference image and motion signals.
The model accepts various input types, including audio signals that drive lip and co-speech gestures, video signals that replicate motion from a reference clip, or a combination of both to achieve fine-grained control over different body parts.
ByteDance designed OmniHuman-1 to handle different aspect ratios and body proportions. This flexibility means the model can produce content suitable for various media formats, ranging from digital advertising to immersive gaming environments. The system is built to overcome limitations seen in earlier frameworks that often required strict filtering of training data and struggled with inconsistent body movements.
Examples from OmniHuman-1
Technical foundations and innovations
Diffusion Transformer Architecture
OmniHuman-1 uses a Diffusion Transformer (DiT) architecture. In simple terms, the DiT framework guides the model to gradually refine its output. The model starts with a rough prediction of human motion and then iteratively improves the details until the final video output resembles natural human movement. This process is analogous to an artist sketching a basic outline before adding finer details.
Multimodal Motion Conditioning
A key innovation in OmniHuman-1 is its multimodal motion conditioning. During training, the model incorporates various forms of conditioning signals:
- Audio-Driven Animation: The model processes speech or music to synchronize lip movements and body gestures. This capability allows for natural co-speech gestures and head movements.
- Video-Driven Animation: The system analyzes reference videos to replicate complex motion patterns. Users can provide a clip as a template, and the model reproduces similar movements.
- Multimodal Fusion: OmniHuman-1 also supports combining audio and video inputs. This fusion provides a more robust control over how different parts of the body move, leading to higher accuracy in gesture realism.
Omni-Conditions Training
OmniHuman-1 applies an omni-conditions training strategy that uses both strong and weak conditioning signals. In this context, “strong” conditions (such as precise pose data) provide detailed guidance, while “weak” conditions (such as audio cues) contribute to data diversity. The training ratio is adjusted so that weaker conditions receive higher emphasis, ensuring that the model generalizes well across various input scenarios.
ByteDance explains that this strategy minimizes data wastage. Instead of discarding motion data that does not meet strict criteria, the model optimizes the use of all available data. As a result, it achieves high-quality animations from minimal input, even when the reference image or audio is not perfect. You can explore the published research here.
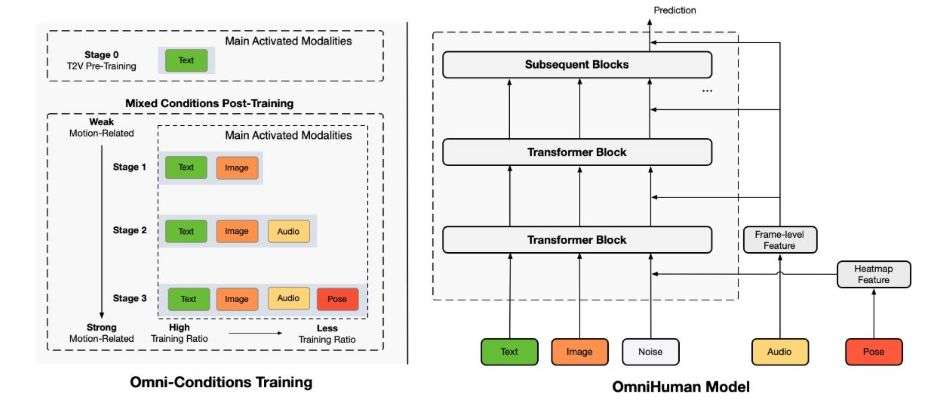
Performance and benchmarking
ByteDance has evaluated OmniHuman-1 against several leading models in the field of human animation. Here are some key metrics from independent tests:
Lip-Sync Accuracy
- OmniHuman-1: 5.255
- Loopy: 4.814
- CyberHost: 6.627
In this metric, higher scores indicate better synchronization between lip movements and audio. OmniHuman-1 shows competitive performance, delivering a realistic match between speech input and animated facial expressions.
Fréchet Video Distance (FVD)
- OmniHuman-1: 15.906
- Loopy: 16.134
- DiffTED: 58.871
FVD measures how closely the generated videos resemble real videos. Lower FVD scores indicate higher video quality. OmniHuman-1’s score demonstrates that its outputs are visually consistent with natural human motion.
Gesture Expressiveness (HKV Metric)
- OmniHuman-1: 47.561
- CyberHost: 24.733
- DiffGest: 23.409
Gesture expressiveness reflects the degree of natural and varied body movements. OmniHuman-1 outperforms competing models by delivering more dynamic and natural gestures, which is essential for applications like virtual avatars and character animation.
Hand Keypoint Confidence (HKC)
- OmniHuman-1: 0.898
- CyberHost: 0.884
- DiffTED: 0.769
HKC measures the model’s precision in tracking hand movements. A higher score indicates better accuracy. OmniHuman-1 achieves a top score, making it effective at generating detailed hand interactions, an important aspect of full-body animation.
Training Data and Scalability
OmniHuman-1 was trained on nearly 19,000 hours of video content, ensuring that it has seen a wide variety of human movements. This extensive training data contributes to the model’s ability to generalize across different body types, poses, and motion scenarios. The scalable training strategy further allows it to produce high-quality animations even when provided with minimal input.
Real-world applications and use cases
OmniHuman-1 is not just a technical achievement—it also has practical applications across several industries. Here are some common use cases reported by early adopters and industry experts:
Virtual Avatars and Digital Influencers
Digital media companies and social platforms can use OmniHuman-1 to create realistic animated avatars from a single reference image. These avatars can engage audiences by mimicking human gestures and expressions, making them valuable for marketing campaigns and social media content.
AI-Driven Character Animation
In the entertainment and gaming industries, OmniHuman-1 enables the creation of lifelike characters with natural motion. Game developers and filmmakers can generate full-body animations without the need for motion capture, reducing production costs and speeding up the creative process.
Digital Storytelling and Educational Content
Content creators use OmniHuman-1 to produce digital storytelling videos that require realistic human animations. Educational institutions and online learning platforms may adopt the technology to create engaging lectures or tutorials featuring animated characters that convey complex information through dynamic visual storytelling.
Virtual and Augmented Reality
OmniHuman-1’s ability to adjust aspect ratios and body proportions makes it suitable for virtual reality (VR) and augmented reality (AR) applications. Developers can generate customized video content that fits different screen formats, enhancing the immersive experience in VR/AR environments.
Editing and Enhancing Existing Videos
The model can also edit existing videos. It can modify human motion in pre-recorded clips, allowing for post-production adjustments. This capability is valuable in scenarios where minor corrections or updates are needed without re-shooting the entire video.
Conclusion
ByteDance’s OmniHuman-1 represents a substantial technical advancement in the field of AI-driven human animation. The model uses a Diffusion Transformer architecture and an omni-conditions training strategy to fuse audio, video, and pose information. It generates full-body videos from a single reference image and various motion inputs, addressing challenges in motion realism, gesture accuracy, and adaptability.
Technical innovations such as multimodal motion conditioning and adjustable reasoning levels allow OmniHuman-1 to perform at a high level. Benchmark evaluations show that the model delivers competitive lip-sync accuracy, excellent gesture expressiveness, and strong hand keypoint confidence. These metrics confirm that OmniHuman-1 generates realistic human animations that are visually consistent and dynamic. However, those “scary good” videos can be used for malicious purposes to create hyper-realistic deep fakes, used by bad actors in scams or blackmailing.
The model’s flexibility and scalability enable it to support a wide range of applications—from virtual avatars and character animation in games to digital storytelling and educational content creation. Moreover, its ability to edit existing videos further extends its utility in post-production workflows.
For professionals working in media, entertainment, and digital communications, OmniHuman-1 offers a powerful tool to enhance productivity and creativity. As AI-generated video technology evolves, tools like OmniHuman-1 set a new standard in generating lifelike human animations from minimal input. By bridging the gap between static images and dynamic video content, OmniHuman-1 transforms how realistic animations are produced, marking a significant step forward in the AI animation landscape.
In summary, OmniHuman-1 provides a robust solution to longstanding challenges in human animation. Its technical strengths, as evidenced by competitive benchmarks and practical applications, make it a valuable asset for those looking to harness AI in digital media production. ByteDance’s focus on multimodality and scalable training paves the way for further advancements in the field, offering creators and developers an efficient, cost-effective way to generate realistic human videos.