The Power of AI Data Analytics: Unlocking the Secrets of Big Data
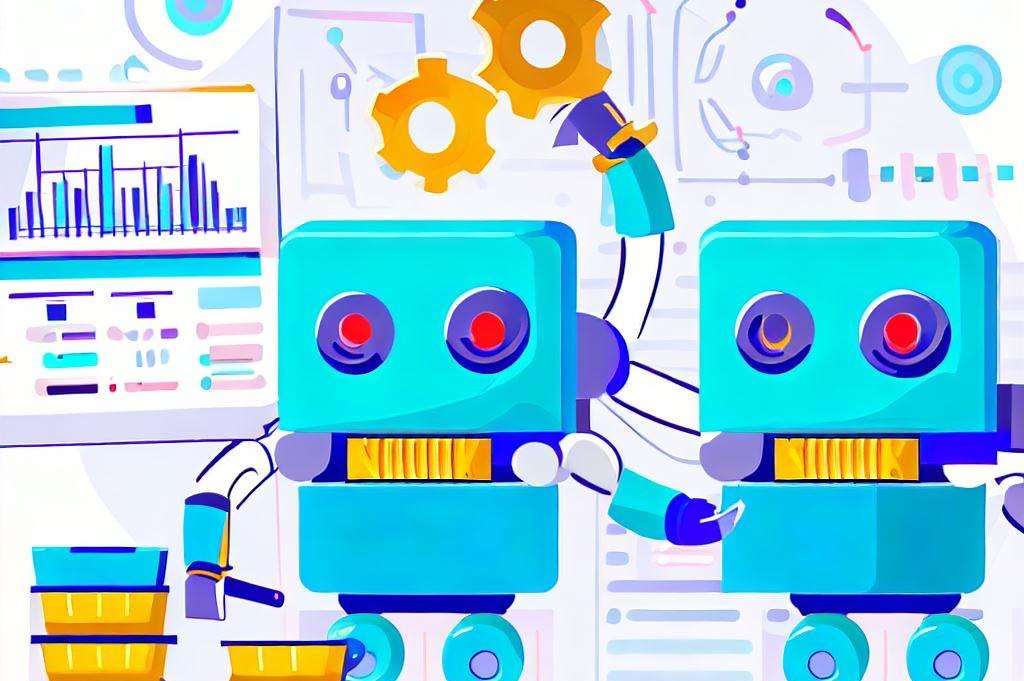
The field of artificial intelligence (AI) and machine learning (ML) has seen rapid advancements in recent years, impacting all types of businesses and providing numerous benefits to advertising and marketing, with one of the most significant developments is AI data analytics
With businesses generating vast quantities of data, AI data analysis enables organizations to make sense of the information in a manageable way, providing valuable insights for marketers when targeting specific customers and empower businesses to filter potential leads and allocate resources to focus on the most profitable customers.
An excellent example of the use of AI in advertising is Google Ads, which uses AI and ML to understand what products interest users. As a result, users see relevant ads that align with their preferences. Additionally, Artificial intelligence analytics is crucial in making sense of the vast quantities of Big Data now available. Traditional data extraction and intelligence tools have limitations, but new AI Analytics tools make it practical and possible.
In summary, with the increasing amounts of data generated every day, businesses require a different approach to turn this data into useful information. This process requires the advanced capabilities of AI, which can make sense of unstructured data, such as audio, video, and image files.
AI data Analytics: How to sort out your data?
Understanding the 3Vs of Big Data Analytics
Data scientists typically define Big Data in terms of the 3Vs: Volume, Velocity, and Variety. Volume refers to the amount of data generated by various sources, while Velocity refers to the speed at which data is generated. Variety, on the other hand, pertains to the different types of data included in the overall collection of data, such as structured, semi-structured, or unstructured data. When analyzing data, it’s essential to consider these 3Vs, particularly in determining the capabilities of a data analysis system.
- Volume – The amount of data generated
- Velocity – The speed at which data is generated
- Variety – Type of data collected
In the field of data analytics, data analysis is the process of examining data and drawing patterns from it. It encompasses both descriptive analysis, which describes what’s happening, and predictive analysis, which predicts what will happen in the future based on current and past activities.
Many businesses face challenges in accessing the data related to their business. Suitable data is often readily available, but companies lack the tools to analyze it into a usable form. Moreover, data may be scattered across multiple sources, requiring businesses to gather all necessary data and convert it into a common form before conducting data analysis.
The Different Types of Data Analytics and their Purposes
According to Gartner’s Ascendency Model, there are four main types of data analytics, namely descriptive, diagnostic, predictive, and prescriptive. Each type serves a specific purpose, with some focusing on identifying patterns in past data, others analyzing the causes of past events, and still, others predicting future outcomes or prescribing actions to achieve a particular goal.
- Descriptive analytics involves summarizing and visualizing past data to provide insights into what happened.
- Diagnostic analytics aims to determine why things happened by analyzing past data in detail.
- Predictive analytics uses machine learning algorithms to forecast future outcomes based on patterns found in past data.
- Prescriptive analytics takes the predictions generated by predictive analytics and recommends actions to achieve specific goals.
By understanding the different types of data analytics, organizations can better leverage their data to drive business growth and success.
Technology Behind Data Analytics: Seven Distinct Technologies
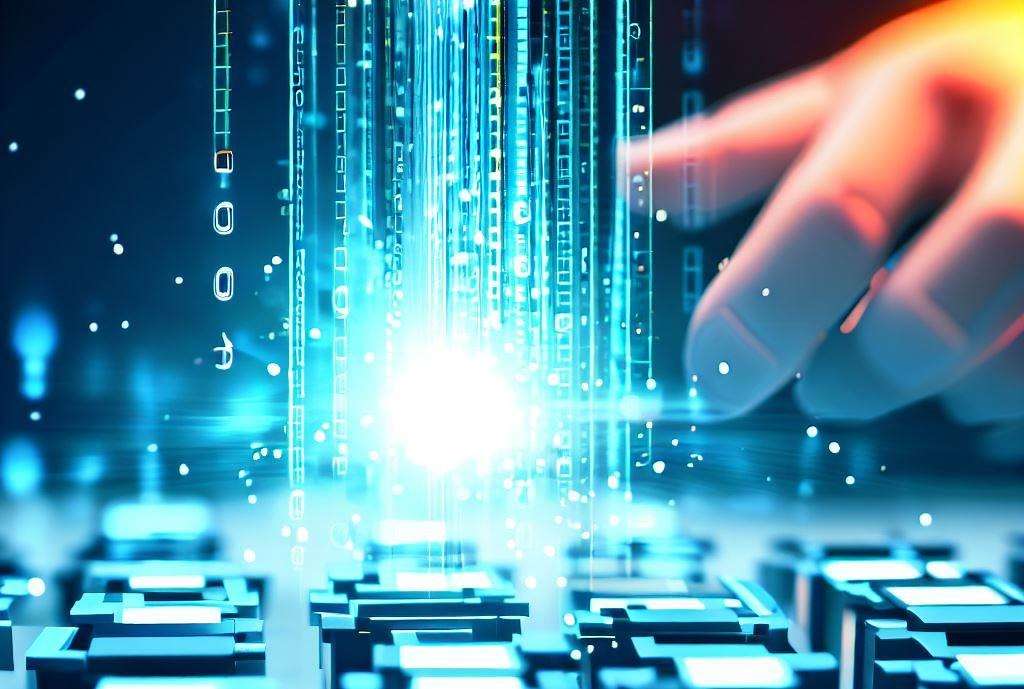
Data analytics and Big Data encompass seven distinct technologies. These include Predictive Analytics, which utilizes statistical algorithms to predict future results based on historical data, Hadoop, a software framework that processes and contains vast amounts of data, and Data Mining, which analyzes vast quantities of data to uncover patterns and connections. Other technologies include Machine Learning, Text Mining, In-memory Analytics, and Data Management, which all contribute to improved data analysis and organization.
AI Data analysis: Changing the Marketing Industry forever
The integration of AI has revolutionized the marketing industry by streamlining data analysis. AI can efficiently process vast amounts of data, automate complex processes, personalize content, and make predictions with unprecedented accuracy. Using machine learning, AI can learn and analyze data independently, significantly reducing the need for human input in traditional data analysis.
The primary differences between traditional data analysis and AI data analysis lie in capacity and computer independence. AI can handle substantially more data and work at superhuman speed to achieve better results. Additionally, AI-based systems can learn and work independently, freeing up staff to focus on other high-priority tasks.
AI Data analytics you can implement in your business
Akkio
Akkio, an AI-powered predictive modeling and decision support platform, has empowered me to automate complex and time-consuming tasks. Its user-friendly interface allows users without a data science background to build custom machine learning models. Whether for predictive maintenance, fraud detection, or customer churn prediction, Akkio integrates seamlessly with popular business applications and data sources.
Akkio
Performance Scores
Pricing
Pros
Akkio's machine learning capabilities allow for advanced data analysis and automation, potentially saving time and effort.
The platform offers integrations with various third-party tools and services, allowing for more flexibility and customization.
Akkio provides templates and pre-built models for common use cases, making it more accessible to users without extensive machine learning experience.
The platform allows for easy deployment and scaling of machine learning models.
Cons
While Akkio offers pre-built models, creating custom models may require significant machine learning expertise.
The platform may not offer as many advanced features or options as other machine learning platforms.
Akkio may not be as well-known or established as other players in the machine learning market.
Screenshots
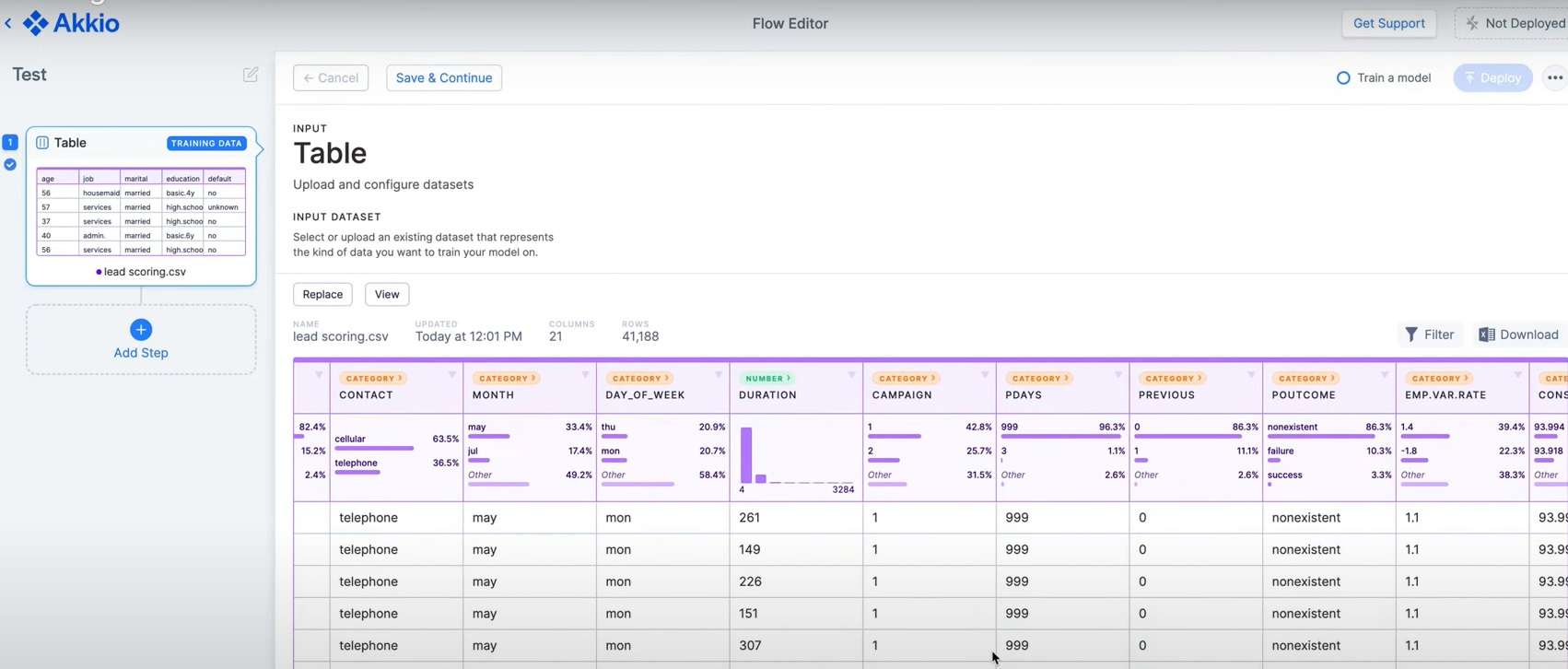
Main Akkio benefits:
- Streamlined and automated data analysis: Akkio makes it easy to analyze large datasets and extract valuable insights quickly, without the need for extensive manual analysis.
- Customizable AI models: The platform allows users to create their own custom AI models using a drag-and-drop interface, without requiring extensive programming knowledge.
- Powerful automation capabilities: With Akkio, users can automate a wide range of data-related tasks, including data cleaning, transformation, and analysis, reducing the time and resources required for these tasks.
- Improved accuracy and efficiency: By leveraging AI and machine learning, Akkio can provide more accurate insights and predictions, while also reducing the risk of errors that can occur with manual analysis.
- Flexible integration options: Akkio can be integrated with a wide range of data sources and tools, including CRMs, databases, and cloud storage services, making it easy to use in a variety of settings.
H2O.ai
H2O.ai is a leading open-source platform for machine learning and artificial intelligence. It provides a user-friendly interface for data scientists and analysts to build, train, and deploy machine learning models at scale
Future of Data Analytics – Embrace the change and stay ahead of the curve
AI data analytics have become a game-changer for businesses, offering them the opportunity to process vast amounts of data and uncover valuable insights and trends. With machine learning, firms can optimize their systems and provide customized service to their customers, enhancing the overall customer experience.
Facial detection and recognition software can help businesses personalize marketing to individuals, while intelligent automation software can adapt marketing messages based on consumers’ emotional reactions. By combining historical customer data, complex algorithms, natural language processing, and emotional analysis, businesses can improve customer service and better predict customer needs.
That’s why, with a large amount of information, best practice in collecting data from customers, it’s to employ a first party data strategy. This gives businesses more control over the privacy of their customers.
Practical examples AI Data Analysis in Business
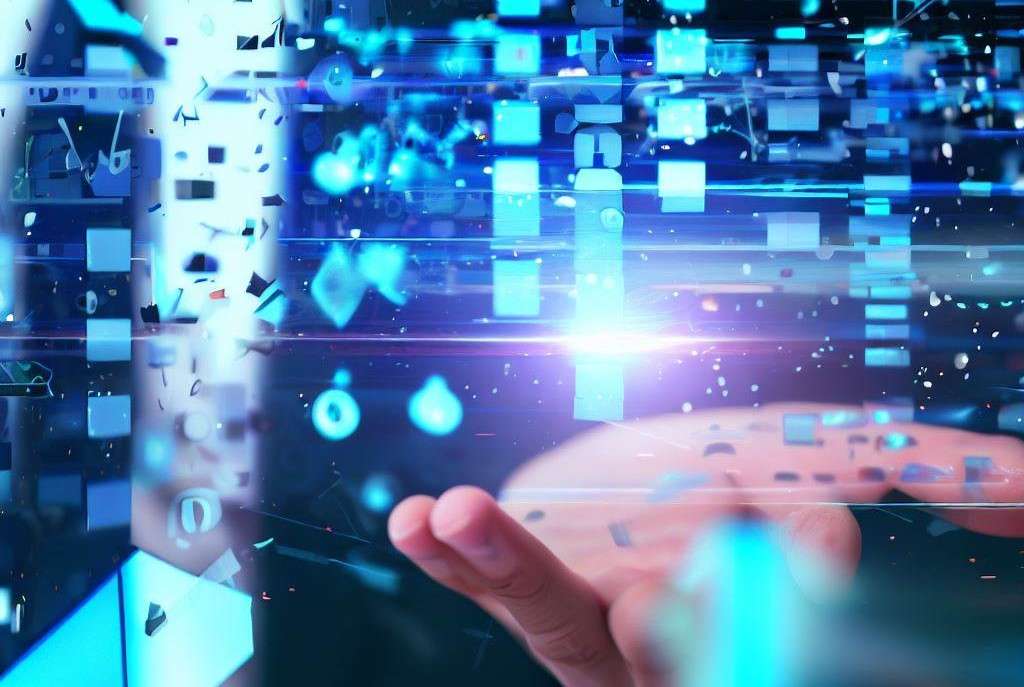
Walmart is one such example of a business utilizing AI data analytics to its advantage. They use artificial intelligence models to predict the optimal inventory mix for any store on a given day, taking into account weather information to reflect customer demand better. Walmart also employs AI smart pricing, using Wi-Fi-enabled cameras to adapt prices in real-time to reduce spoilage and waste, leading to a 30% sales increase in the department.
AI can also revolutionize the way businesses gather product and consumer insights by leveraging unstructured data analytics to track consumer behavior and identify unmet needs. Text analytics can provide insight into customer sentiment, conversation trends, and other valuable information, allowing businesses to anticipate customer needs and market trends better.
Predictive and prescriptive data analytics enable organizations to gain insights into customers’ needs and preferences before they become apparent. This facilitates informed decision-making in areas such as inventory management, customer service, operational efficiency, and customer retention. By leveraging data analytics, businesses can optimize their operations, minimize wastage, and improve their bottom line, ultimately increasing their customer lifetime value.
Learn more about AI automation in business and how data can support the seamless implementation of artificial intelligence in business processes and marketing.
Conclusion – Implementing effective AI analytics tools in your business will improve ROI and productivity
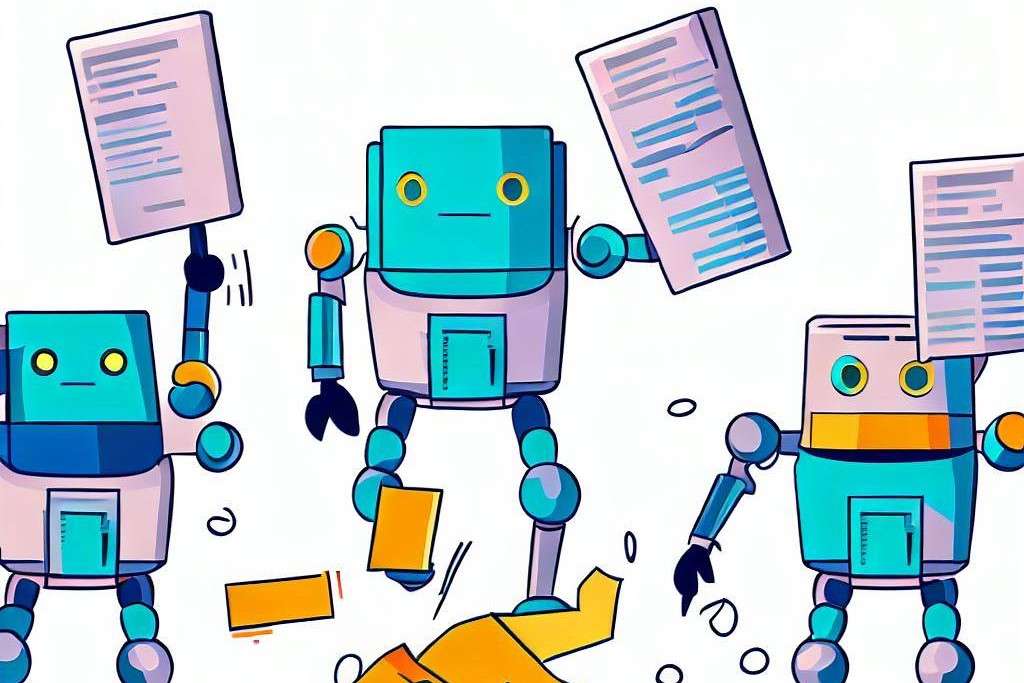
In conclusion, AI data analytics has revolutionized the field of data science by providing unmatched speed, scale, and precision in monitoring and analyzing large datasets. By automating tasks that would normally be time-consuming for human analysts, businesses can save time and resources while improving their operational efficiency. Another significant benefit of Artificial intelligence analytics is its ability to provide objective insights, free from human biases and assumptions. As a result, businesses can make data-driven decisions with more confidence and accuracy. As technology continues to advance, the role of AI data analytics will only become more prominent, leading to even more significant advancements in the field.