Cracking the Code: Open Source AI vs. Closed Source AI – Exploring Pros & Cons
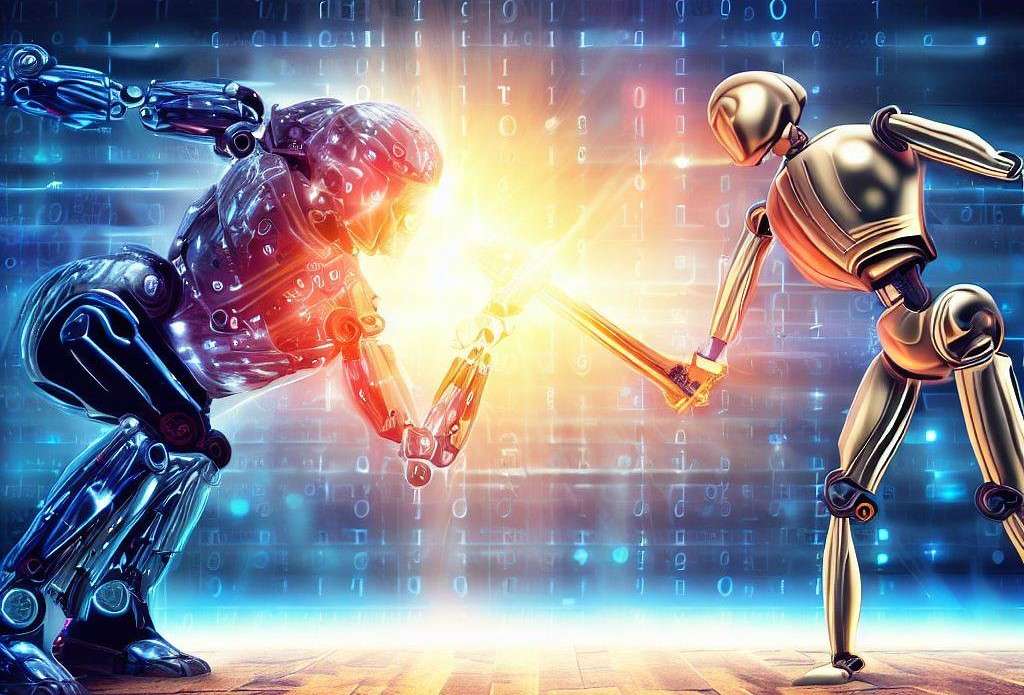
In the world of AI technologies, a battle for control is underway, captivating the attention of researchers and industry giants alike. Not only technology giants are at war, but also the underlying technology of Open Source AI vs Closed Source AI has came to the spotlight. The call for a temporary halt on AI experiments, spearheaded by visionaries like Elon Musk and prominent ML researchers, reflects the gravity of the situation.
Recent times have witnessed a surge in the release of numerous large AI models, continually refined through the infusion of additional data. This fierce competition among companies to emerge as the leading AI provider has resulted in razor-thin differentiators between them. As OpenAI ‘s ChatGPT and OpenAI Playground takes the lead in the race for Artificial Intelligence dominance by democratizing the access for the masses, it has sparked a landscape of innovation, collaboration, and occasional controversy. In this ever-evolving environment, the quest for control intensifies, raising the stakes to unprecedented heights.
When it comes to AI development, there are two main approaches: open source AI and closed source AI. In this blog post, we will delve into the major differences between these two models and explore the spectrum that exists between them.
Ilya Sutskever, co-founder and chief scientist of OpenAI, explains his general approach to deciding whether an AI model should be open- or closed-source. Watch more from Standford E-Corner here.
Open Source
AI Open source AI refers to AI frameworks and tools that are developed and shared openly, allowing anyone to access, use, modify, and distribute the code. The benefits of open source AI are numerous. It promotes collaboration, transparency, and customizability. Developers can work together, share ideas, and contribute to the improvement of AI technologies. Popular examples of open source AI frameworks include TensorFlow and PyTorch.
Recently, Cerebras made headlines by introducing Cerebras-GPT, claimed to be the first fully open-source large language model optimized for computing. Emphasizing the importance of open access to advanced models, Cerebras released Cerebras-GPT under the Apache 2.0 license, enabling royalty-free utilization for both research and commercial applications.
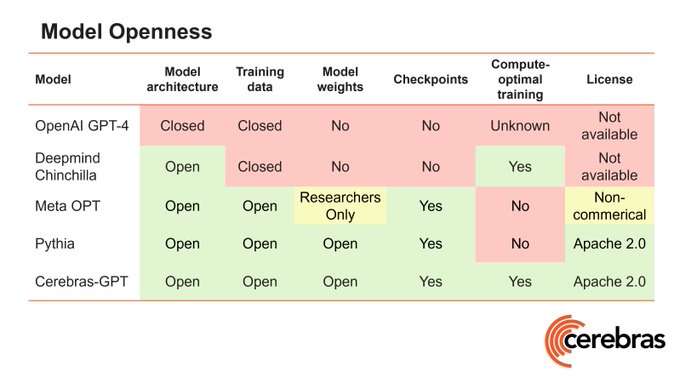
Closed Source
AI Closed source AI, on the other hand, involves proprietary technologies that are developed and controlled by specific companies or organizations. The source code is not publicly accessible, limiting the ability to modify or distribute it. Closed source AI offers advantages such as intellectual property protection and specialized support. However, it lacks the transparency and community-driven development found in open source AI. Prominent examples of closed source AI platforms include Open AI GPT, IBM Watson and Microsoft Azure AI.
Open Source AI vs Closed Source AI
When considering open source versus closed source, several factors come into play. In terms of performance and functionality, both models can deliver impressive results. However, open source AI often benefits from the collective expertise of a larger community, resulting in faster innovation and a wider range of features.
Accessibility and cost are also important considerations. Open source is typically free to use, making it more accessible to individuals and small organizations. Closed source AI platforms may require licensing fees or subscriptions, making them more suitable for larger enterprises with specific requirements and budgets.
Privacy and security implications differ between the two models as well. Open source AI fosters transparency, allowing users to inspect the code and identify potential vulnerabilities. Closed source AI, while providing robust security measures, may raise concerns about data privacy and the handling of sensitive information.
The Spectrum between Open Source AI and Closed Source AI
It’s important to recognize that the open source and closed source models represent two extremes on a spectrum, with varying degrees of openness and proprietary control in between. Some organizations adopt a hybrid approach, using a combination of open and closed source technologies to meet their specific needs. Additionally, certain open source projects may incorporate closed source elements for commercialization purposes.
Licensing models play a significant role in determining the level of openness. Some open source licenses, such as the GNU General Public License (GPL), require derivative works to be released under the same license, ensuring continued openness. Other licenses, like Apache License, allow for more flexibility in the use and distribution of code.
What can work best for my needs?
Presently, open-source and closed-source models exhibit comparable performance levels as they rely on similar datasets, encompassing the vast expanse of the open web. However, a crucial question arises: Can open-source models, relying solely on publicly available data, maintain competitiveness against closed-source models equipped with proprietary datasets? The answer lies in the specific use case at hand.
In specialized domains such as medical diagnoses, pharmaceutical development, advanced scientific research, and fraud detection, where highly specialized skills are required, closed-source models are likely to prevail. These areas entail substantial costs for acquiring training data, rendering them less likely to be included in open-source models.
Conversely, open-source models can sufficiently compete in areas where expertise is not the primary requirement. Tasks such as customer service support, normal conversation, text and speech adaptation, text summarization, stock photo creation, and email generation are well-suited for open-source models. Reinforcement learning with human feedback (RLHF) approaches can augment these domains. Notably, incorporating additional data into open-source models is easier compared to proprietary data, as individual units of data hold lower value. In less specialized realms, open-source models are poised to excel, maintaining a satisfactory level of performance without falling behind.
Ethical considerations and safeguarding AI
In the evolving landscape of AI, the deployment of AI systems lacks standardized norms, leading to debates about responsible practices. While open-source and closed-source models both have their merits, ethical considerations are paramount. Safety research, safeguards, and responsible licenses are necessary for all systems, regardless of their openness. Technical provisions alone are not enough, and policy frameworks, content moderation, and knowledge-sharing among labs play crucial roles in ensuring ethical AI practices. The gradient framework offers a nuanced approach to analyze access and make informed release decisions. Ultimately, it is essential for labs to evaluate systems before deployment, manage risks post-release, and prioritize ethical considerations throughout the AI development process.
Conclusion
As AI continues to evolve, it’s crucial to consider the strengths and limitations of each model. Organizations must evaluate their requirements, budget, privacy concerns, and desired level of community involvement. Moreover, the spectrum between open source and closed source AI provides opportunities for innovation, hybrid models, and licensing adaptations.
Ultimately, the future of AI development lies in striking a balance between openness and proprietary control, ensuring that the technology benefits humanity as a whole. By embracing the strengths of both open source and closed source AI, we can harness the full potential of AI to solve complex problems and drive innovation in the digital age.