How to build an AI Startup in 2024: What they don’t teach you in Business School
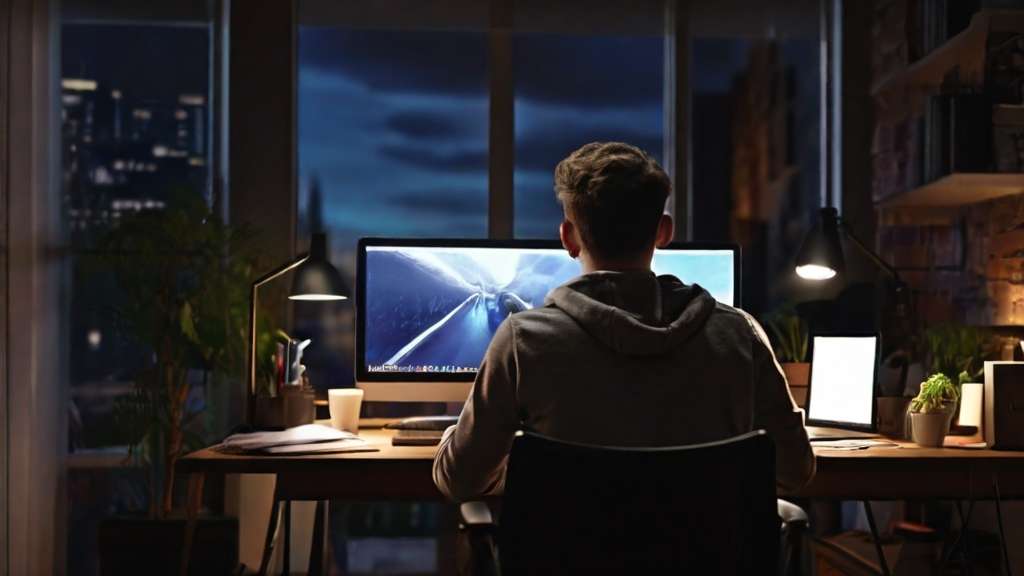
Building a Successful AI Startup in 2024 takes more than a good idea
The rapid evolution of artificial intelligence (AI) has fueled a boom in AI startups, attracting nearly $50 billion in venture capital funding in 2023 alone. With the global AI market set to exceed $1 trillion by 2025, the potential for new AI-driven businesses is vast. Yet, this landscape also demands careful navigation. To thrive, AI startups must solve real problems, overcome technical hurdles, and compete for funding. Keep reading if you want expert tips on how to build an AI Startup in 2024.
Key Takeaways
- Find the pain point: Don’t build AI for the sake of it. Identify a critical, unsolved problem that customers are willing to pay to fix.
- Validate relentlessly: Talk to potential customers early and often. Get feedback on low-code prototypes before sinking time and money into full development.
- Become the expert: Specialize in solving problems within a specific industry. This helps you stand out from generic AI solutions.
- Build a balanced team: Seek co-founders or early hires who complement your skills. You need both technical AI expertise and business savvy.
- Embrace ethics: Build trust and avoid long-term risks by designing your AI startup with fairness, transparency, and accountability from the beginning.
- Distribution matters: Don’t fall into the “build it and they will come” trap. Invest in marketing and sales strategies from the start.
- Stay adaptable: The AI landscape changes rapidly. Be ready to pivot your approach or refine your product based on market feedback – that’s how to build an AI Startup.
“The best way to predict the future is to invent it.”
Alan Kay – 1971
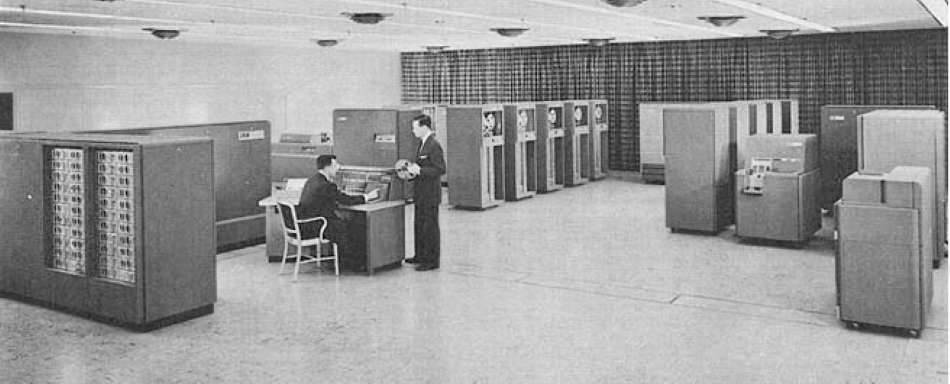
Early on, AI startups focus on prototyping, customer discovery, and building core AI models. Bootstrapping and seed funding are common, fueling the drive for product-market fit. Success hinges on relentless customer focus and validation of technical feasibility. These early wins pave the way for the scaling phase to come.
2023 has set the stage for explosive Development and AI startup creation
2023 has set the stage and propelled Artificial Intelligence into mainstream by captivating the human imagination. There is excitement, there is fear and most importantly there is innovation and increased infrastructure to build our future. Moreover, AI can be your business consultant that can guide you on how to build an AI startup!
Mainstream Implementation and Scaling from 2023 and Beyond
2023 marked a turning point for AI adoption. Gartner predicts 75% of enterprises will operationalize AI by the end of 2024, signifying a major shift. This widespread integration offers both challenges and immense opportunities for scaling AI startups. Companies at this stage refine AI models, invest in robust infrastructure, and aggressively expand their market presence.
Forerunners will see significant rewards. AI in retail, for example, could boost productivity by 30% through tailored experiences (according to BCG) and optimized supply chains. However, hurdles remain: the intense competition for AI talent, ethical considerations, and ensuring model accuracy over time.
Several crucial factors are fueling this transformation: Breakthroughs in powerful AI models now offer unprecedented problem-solving capabilities, while a focus on explicability helps users understand and trust AI-powered systems, fostering broader adoption.
The continuous growth of big data supplies the fuel for training increasingly sophisticated AI models, and the increasing accessibility of AI infrastructure through cloud platforms and specialized tools lowers the barrier to entry for innovation. These trends, combined with a steadily expanding talent pool, create a fertile environment for the next wave of AI-driven breakthroughs.
Pain Points and Opportunities for Forerunners and Innovators of AI Startups
The AI revolution promises great rewards for those who seize the moment. Early movers grapple with the field’s rapid pace, potential model drift, and the need to build trust through transparency. Strategic focus on MLOps, ethical AI, and adaptability will set leaders apart. Alongside technical prowess, strong partnerships, effective go-to-market strategies, and substantial funding rounds will propel AI startups into industry dominance.
Types of AI Startups – A simple classification
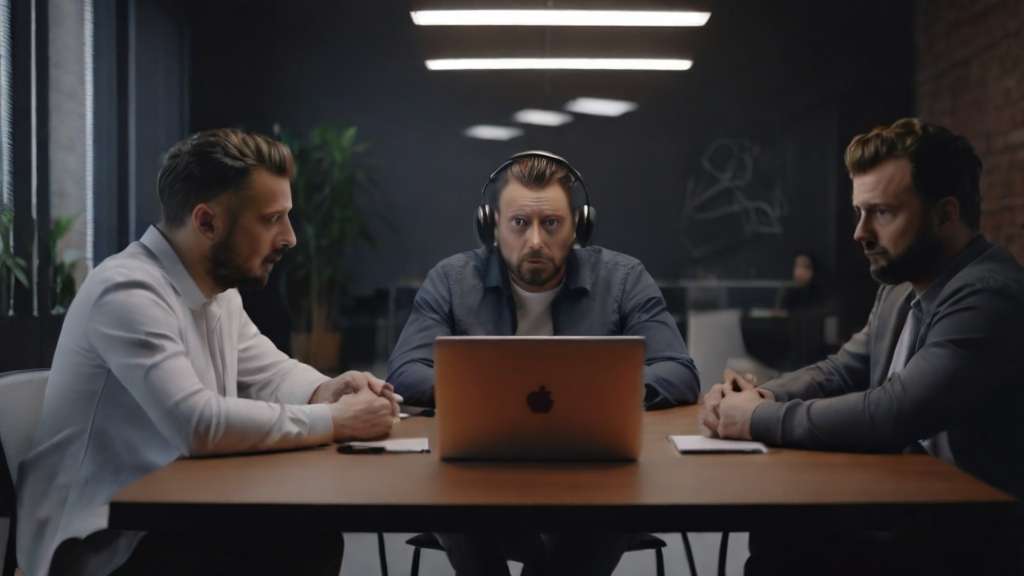
- Solo/Micro-Startups: 1-5 people, early prototyping, testing ideas, little revenue.
- Bootstrapped Startups: Small teams, self-funded often through client work, may have seed funding, working toward product-market fit.
- VC-Backed Scaleups: 10+ employees, significant venture funding, ready for hypergrowth.
- AI Unicorns: Large companies, multi-national operations, often exited from VC through IPO or acquisition. Top example that took the world by storm is Open AI, the giant powering a large portion of SaaS AI Start ups.
Top AI Startup Business Models: Finding Your Niche
Let’s look at popular business models gaining traction within the AI landscape and choose your approach on how to build an AI Startup:
Micro-SaaS
- Benefits:
- Quick to market due to focused problem-solving
- Lower customer acquisition costs through targeted solutions
- Recurring revenue stream through subscription models
- Ability to scale vertically within a niche by upgrading features
- Practical Applications: Grammar and writing helpers, image editing & enhancement, social media analytics plugins, SEO automation tools
- Opportunities: Huge potential in identifying underserved niches with acute pain points AI can alleviate. Integrations with larger platforms increase reach.
- Challenges: Risk of market saturation in popular niches, pricing pressure due to ease of use, churn risk if competitors emerge with better value propositions.
AI Consultancies
- Benefits:
- Rapid revenue generation through project-based and hourly billing
- Ability to leverage existing AI expertise without building products from scratch
- Deep partnerships with clients foster long-term relationships
- Practical Applications: AI strategy development, algorithm selection & customization, data preparation & pipeline design, system deployment & monitoring
- Opportunities: Growth tied to the surge in mainstream AI adoption, potential for specialization in specific industries or verticals.
- Challenges: Maintaining a talent pool with diverse AI skills, competition from large systems integrators, staying ahead of rapidly changing AI tools.
Industry-Specific Platforms
- Benefits:
- Solve critical pain points with high willingness-to-pay from customers
- Reduced competitive pressure due to niche focus
- Potential to become the standard platform through deep domain knowledge
- Practical Applications: Fraud detection in finance, predictive maintenance in manufacturing, drug discovery in pharmaceuticals, patient diagnostics in healthcare
- Opportunities: Expansion to adjacent use cases within an industry, building complementary services around the platform, potential for strategic partnerships.
- Challenges: Slower revenue growth initially due to longer sales cycles, need for industry expertise alongside AI talent, data quality and availability can be a bottleneck.
Data Monetization
- Benefits:
- Potentially massive upside if collecting unique, high-value data
- Multiple revenue streams (marketplaces, APIs, custom reports)
- Can be a strategic moat against competitors
- Practical Applications: Weather pattern data, specialized medical image datasets, location data aggregated for retailers, financial transaction data
- Opportunities: Partnerships with entities lacking data collection capabilities, advanced data cleaning and enrichment services, bespoke analysis for customers
- Challenges: Significant upfront investment in infrastructure and data pipelines, legal and regulatory compliance, may need substantial VC backing.
ML Model Development & Licensing
- Benefits:
- Capital-efficient for specialized research teams
- Low customer acquisition costs with a focus on B2B licensing
- Attractive acquisition target for larger tech companies seeking to expand AI capabilities
- Practical Applications: Novel NLP models, computer vision algorithms, recommendation engines, reinforcement learning systems
- Opportunities: Breakthrough research leading to widely adopted models, securing patents, targeting underserved industries where off-the-shelf models perform poorly
- Challenges: Intense competition from academia and Big Tech, sales cycle dependent on client R&D capabilities, risk of commoditization with time.
Critical Tech Stack Decisions when building a successful AI Startup
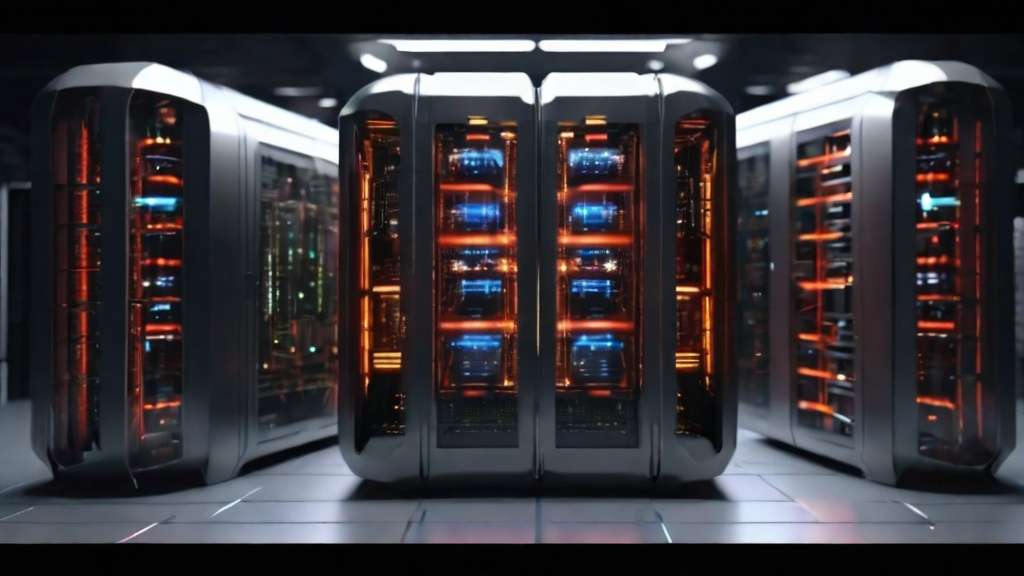
Choosing the right technology foundations influences the agility and success of your AI startup in 2024 and beyond. Ask yourself:
- Open Source Leverage: Will you prototype with frameworks like TensorFlow, PyTorch, or Hugging Face for fast iteration? Open-source is a powerful tool where you get more control
- Custom Models: Do you have the ML expertise to build in-house models for differentiated performance and defensibility?
- Foundation Models: How will you leverage GPT-4 or Anthropic Claude, or similar large language models (LLMs) to bootstrap product capabilities?
- Build on Top of LLMs: Does your focus center on fine-tuning LLMs and specializing them further?
- MLOps Tooling: How early will you invest in MLOps for production-grade AI?
How to build an AI Startup: Stages of growth
Building any successful business isn’t about a single brilliant idea; it’s a systematic journey of validation and scaling. Here’s a breakdown of typical growth stages of AI Startups:
Phase 1: Prototyping & Customer Discovery
- Focus: Rapid experimentation to explore core value propositions and technical feasibility.
- Low-Code Tools: Leverage platforms for quick MVP mockups to gather user feedback.
- Customer Interviews: Talk to potential users to identify real pain points and validate willingness to pay for solutions.
- Market Research: Analyze competitors and trends to refine your niche and messaging.
- Outcome: Gaining initial signal on problem/solution fit, informing product direction or encouraging pivots.
Phase 2: Private Beta Launch
- Focus: Building out functionality, product refinement, and initial go-to-market testing.
- Team Growth: Early hires for development, data science, UI/UX, and product management.
- Data & Model Pipelines: Formalizing processes for data collection, cleaning, and model training.
- Pre-Seed/Seed Funding: Raising capital (if needed) to accelerate development and support a small team.
- Outcome: Achieving a working product with a limited set of early customers for rigorous testing, pricing model validation, and gathering testimonials.
Phase 3: Public Launch & Scaling
- Focus: Gaining broader traction, solidifying product-market fit, and growth optimization.
- Marketing & Sales: Investing in paid acquisition, content marketing, building an effective sales pipeline.
- Pricing Finalization: Establishing sustainable pricing tiers based on beta learnings.
- Team Expansion: Hiring across engineering, customer success, sales, and operations.
- Series A Funding: Significant fundraising round fueled by traction metrics and ambitious growth plans.
- Outcome: Robust product with established market presence, aiming for category dominance through aggressive growth.
Common AI Startup Challenges
You might be an entrepreneurs at the beginning of the journey or a seasoned, tech savvy business owner, potential challenges of building a successful AI Startup remains and they are unique. Here are some potential issues you might encounter:
Technical Challenges
- Model Degradation & Drift: Maintaining accuracy over time requires active monitoring and re-training.
- Data Quality & Bias: Unreliable or biased data can cripple your AI, highlighting a need for rigorous sourcing and bias mitigation.
- Explainability & Trust: Users may be reluctant to adopt AI if they can’t understand its decisions.
- Technical Debt: Rapid iteration can create complex code that’s difficult to scale and maintain.
Business Challenges
- Unclear Value Propositions: AI-for-AI’s-sake is unproductive; focus on solving concrete customer problems.
- Misaligned Technology & Market: Powerful AI features don’t guarantee a viable business model.
- Sales & Distribution: AI products may require specialized sales efforts and deep customer education.
- Hiring Experienced Talent: Finding and attracting AI engineers, data scientists, and leaders is intensely competitive.
Fundraising Challenges
- Limited Revenue: Early-stage AI startups may struggle with aggressive financial projections.
- Future-Focused Vision: Investors need to see the path to tangible results, not just abstract AI potential.
- Lack of Differentiation: Commoditized AI solutions face skepticism and intense competition.
Navigating Challenges
- Prioritizing Customer Understanding: Develop empathy for your userbase and the specific challenges they face.
- Building a Robust Team: Balance technical talent with domain experts, product specialists, and seasoned sales leadership.
- MLOps Best Practices: Invest in MLOps early for production-ready, scalable AI systems.
- Demonstrating ROI: Quantify benefits for customers and focus on real-world outcomes.
- Focus & Execution: Avoid overpromising with multiple products or scattered solutions.
From the Tech Pilot Team: Tips for Your AI Startup Journey
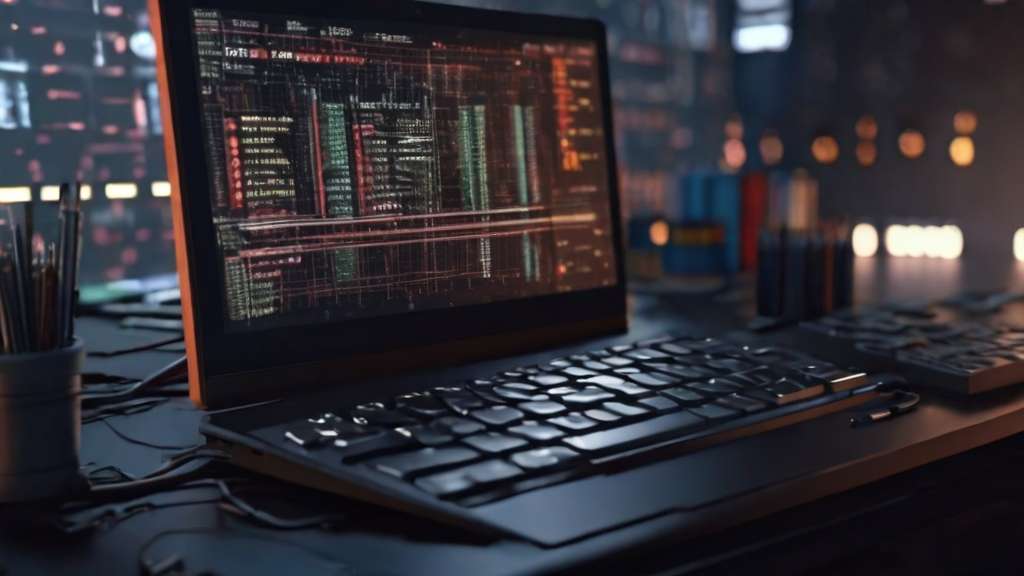
Navigating the fast-paced world of AI startups can be exhilarating but full of hurdles. If you’re struggling with finding the right problems to solve, overwhelmed by technical complexities, or unsure how to turn your AI vision into a thriving business, we’re here to help.
The Tech Pilot team combines years of hands-on experience in both entrepreneurship, startups and AI technology. We’ve faced the same challenges you might be facing now, and through trial and error, we’ve discovered the strategies that truly make a difference. Consider these tips your roadmap to success:
- Chase Real Problems, Not Hype: Ground your solutions in solving actual customer pain points instead of chasing fleeting technology trends.
- Start with a Low-Code MVP: Build quick prototypes to understand the product’s potential and gather early feedback before investing in heavy development.
- Talk to 100+ Customers: Before writing a single line of code, prioritize in-depth interviews and surveys to validate the market need fully.
- Operationalize MLOps Early: Integrate model monitoring, governance, and continuous retraining for robust AI systems in production environments.
- Seek Co-Founders: Balance technical prowess with business acumen and commercialization experience by finding a complementary co-founder.
- Consider Licensing & IP: Explore the potential for licensing your core AI models or algorithms as an alternative or supplemental revenue stream.
- Focus on Vertical Mastery: Become an expert in a specific industry to establish your value proposition and outcompete general-purpose AI tools.
- Emphasize Distribution: Invest in effective marketing channels and sales strategies right from the start; building a great product isn’t enough.
- Embrace Ethical AI: Integrate fairness, transparency, and accountability into your development processes to gain trust and mitigate risks.
Examples of Emerging AI Startups
- Micro-SaaS:
- AI Consultancies:
- DataRobot: Platform and services to help organizations leverage AI across various use cases.
- Industry Platforms:
- Landing AI: AI solutions tailored to quality control and inspection for manufacturing.
- Data Monetization:
- Hugging Face: Hub for pre-trained AI models, datasets, and building a marketplace for monetized access.
The Road Ahead for AI Startups
2024 and beyond present unparalleled opportunities to build AI startups with real-world impact.
Building the next AI unicorn is a demanding journey and with slim chances of success. However, by leveraging the strategies outlined in this guide, you’ll maximize your chances of navigating challenges, seizing opportunities, and creating lasting value through the power of artificial intelligence. There are many nuances of entrepreneurship and we are aware that we only scratched the tip of the iceberg on how to build an AI Startup – Keep learning, keep innovating and keep trying.